Choosing the Right Data Architecture (Part I)
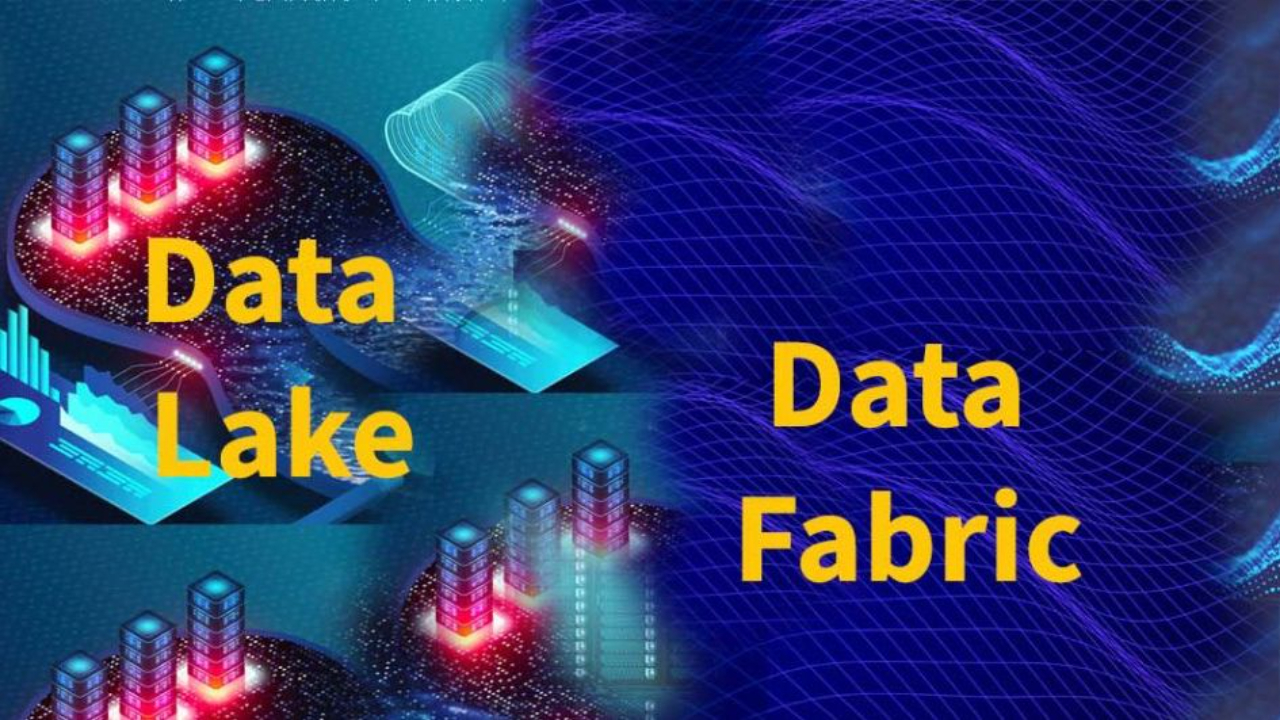
Data growth has been exponential in recent years due to the proliferation of digital devices and the increasing use of the internet, cloud computing, and connected devices coupled with declining cost of storage. With cheaper storage costs, individuals and organizations are storing more data than ever before without worrying about the associated costs. This has led to the creation of massive amounts of data, expanding beyond the OLTP (online transaction processing) & POS (point of sale) systems to also include sources such as: social media posts, emails, videos, images, and other digital content. Additionally, cloud computing providers have made it easier and cheaper to store data remotely, without the need for expensive on-premises infrastructure. Cheap storage costs have also encouraged organizations to retain more data for longer periods, as it is now more cost-effective to do so. This has led to the creation of massive data repositories, which can be mined for insights and used for various applications such as AI and machine learning.
This trend presents both opportunities and challenges for individuals and organizations, as they seek to harness the power of data while also ensuring its responsible use and management. And it all starts from choosing a data storage architecture, which becomes a complex and difficult exercise, due to various tradeoffs involved like:
- Data volume & velocity: It includes both the amount and velocity of data increase on hourly, daily, weekly or monthly basis. Data storage architecture must be scalable and flexible to handle data ingestion and processing.
- Data Variety: It refers to various formats such as structured, semi-structured and unstructured coming from various sources. Data storage architecture must be able to accommodate different data types and integrate the data from various sources.
- Data Governance: Data storage architecture must comply with data privacy laws, regulations, and standards. It must provide data security, access control, and auditing to ensure data integrity and confidentiality.
- Cost and complexity: Data storage architecture can be costly to implement and maintain, and it can be complex to manage. Organizations must balance the cost and complexity of the architecture with the benefits it provides.
- Changing technology landscape: The technology landscape for data storage is constantly evolving, with new technologies and tools emerging frequently. Organizations must keep up with the latest developments to ensure that their data storage architecture remains up-to-date and effective.
Given these choice parameters, common data architectures fall under one of four frameworks- Data Lake, data warehouse, data fabric and data mesh. While all these terms are used to describe architectures for managing and analyzing large volumes of data. However, they differ in their underlying principles and implementation approaches. We can understand brief overview of each and difference among them as follows:
Data lake: A data lake is a centralized repository that stores raw and unstructured data from various sources, such as OLTP, POS systems, IoT devices, social media, and databases, without any transformation or organization. Data lakes are often used for data exploration and advanced analytics since they provide a flexible environment for data scientists to work with the data. However, the lack of organization can make it difficult to find relevant data and lead to data quality issues.
Data warehouse: A data warehouse is a structured repository that stores historical data from various sources in a pre-defined schema for easy querying and reporting. Data warehouses are typically used for business intelligence and decision-making purposes, such as generating reports or analyzing trends. Data warehouses require a lot of planning and upfront work to design and implement the schema, and they can be inflexible if the data changes frequently.
Data fabric: A data fabric is an architecture that allows data to flow seamlessly between different systems, applications, and services. It provides a unified view of data across the organization, regardless of where it's stored or how it's accessed. Data fabric is a relatively new concept that focuses on data integration and interoperability, with an emphasis on data democratization and self-service analytics.
Data mesh: A data mesh is a distributed architecture that decentralizes data ownership and management to enable faster innovation and collaboration. It's based on the principles of domain-driven design, where each data domain is managed by a dedicated team that's responsible for the data's quality, governance, and accessibility. Data mesh aims to reduce the reliance on centralized data teams and provide more autonomy to domain experts.
While, data lake, data warehouse, data fabric, and data mesh are all different approaches to managing data, each with its own strengths and weaknesses. Choosing the right architecture depends on the organization's goals, resources, and data maturity level. I will be covering some of these aspects in Part-II of this article later this week. For more articles like these please like, follow me and share your thoughts.
Stay connected with news and updates!
Join our mailing list to receive the latest news and updates from our team.
Don't worry, your information will not be shared.
We hate SPAM. We will never sell your information, for any reason.